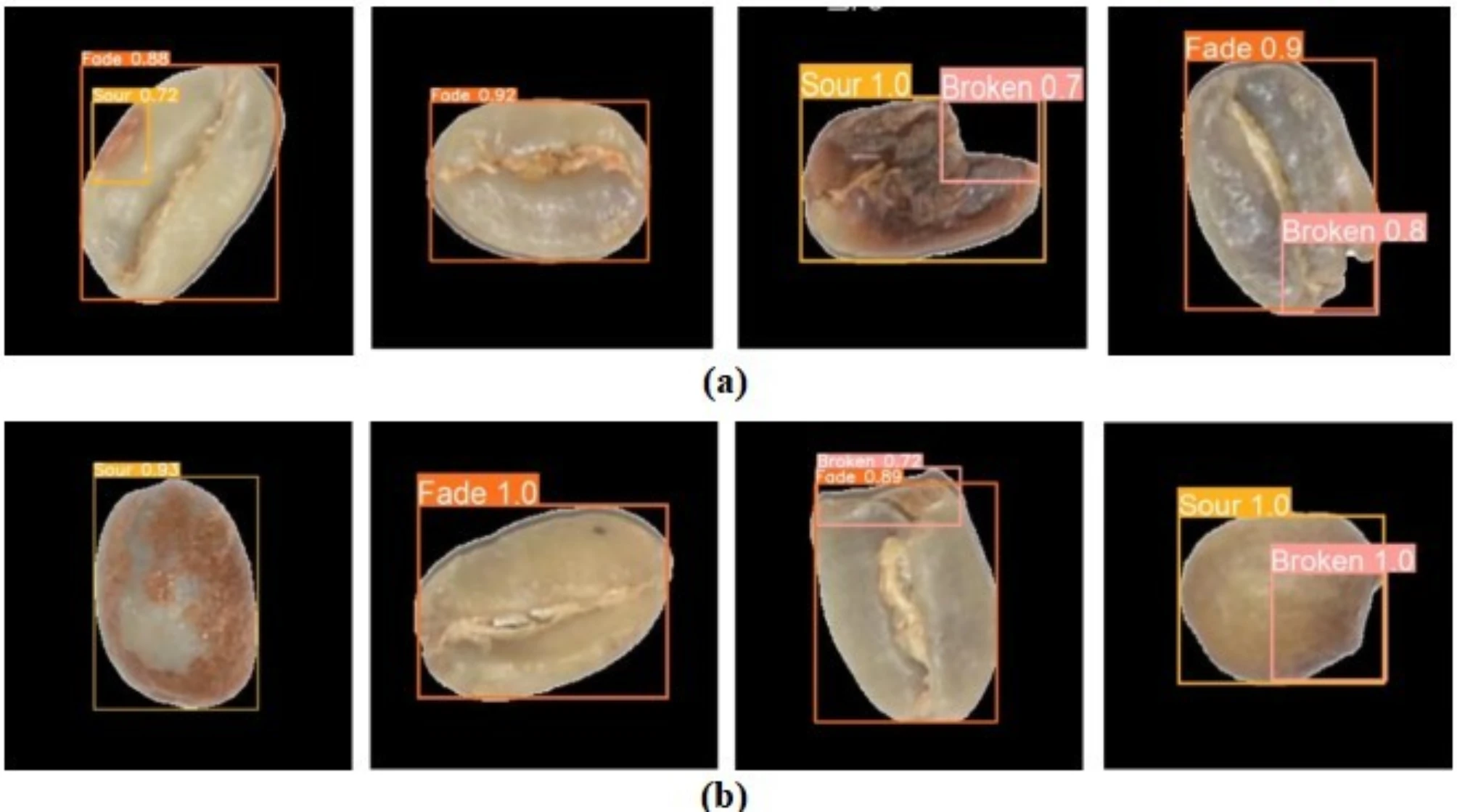
A visible of detection outcomes of a personalized computational mannequin for figuring out espresso defects.. Using this picture is licensed below a Artistic Commons Attribution-NonCommercial-NoDerivatives 4.0 Worldwide License. See the unique right here.
A analysis crew targeted on potential real-world AI options for agricultural producers says it has created a extremely correct computational mannequin for figuring out defects in inexperienced coffees.
The group of scientists from Bangladesh and Japan in contrast a number of variations of personalized YOLO (You Solely Look As soon as) fashions. YOLO is a sort of deep studying algorithm for real-time object detection that’s already in use in functions similar to medical imaging, safety cameras and self-driving vehicles.
The analysis crew stated using YOLO modeling in inexperienced espresso manufacturing would possibly finally profit inexperienced espresso producers and different business actors who’ve relied available sorting for numerous high quality management duties.
“Detecting faulty beans is important for bettering espresso manufacturing processes,” the researchers wrote, including, “particularly in creating international locations like Bangladesh, the place it might considerably enhance the financial system and enhance the livelihoods of farmers.”
As of this writing, there was not less than one machine already in use within the espresso business using AI algorithms for real-time defect detection, the Avercasso CS1 from Taiwan. Its algorithms and {hardware} stay proprietary.
For the brand new open-access examine, the researchers collected greater than 5,000 high-resolution photos of inexperienced espresso beans, whereas figuring out for defects listed as: black beans, damaged beans, pale beans and bitter beans.
These photos have been used to coach six completely different YOLO fashions, every of which was then examined for accuracy.
One custom-modified mannequin, referred to as YOLOv8n, stood above the remainder of the sector when it comes to defect identification accuracy. The mannequin additionally bested different fashions in minimizing false positives and negatives.
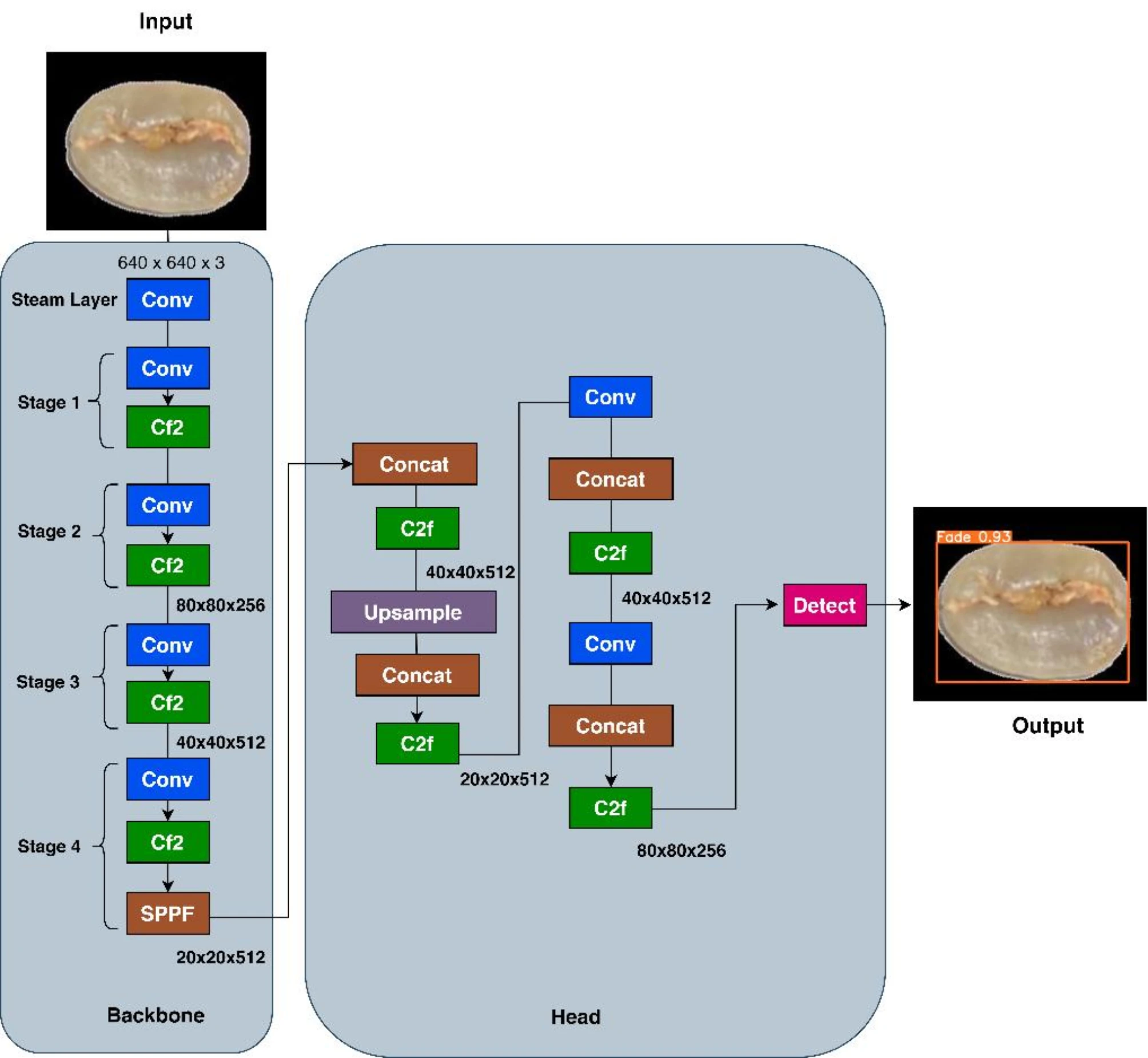
The structure of the personalized YOLOv8n mannequin. This use of this picture is licensed below a Artistic Commons Attribution-NonCommercial-NoDerivatives 4.0 Worldwide License. See the unique right here.
“The proposed custom-YOLOv8n mannequin exhibited superior efficiency throughout all key metrics when in comparison with earlier variations, suggesting its sturdy potential for sensible functions in espresso bean defect detection,” the examine states.
Nevertheless, the researchers additionally famous a number of sensible limitations, together with vital computational sources required to refine the mannequin and an in depth coaching course of.
Moreover, the examine targeted on coffees from a single supply in Bangladesh, that means the efficacy of the fashions could not essentially translate when utilized to various kinds of coffees from completely different international locations or areas.
“Increasing the dataset to incorporate beans from various origins and defect varieties might enhance the mannequin’s generalizability,” the researchers wrote. “Future analysis might additionally combine IoT (Web of Issues) gadgets for real-time monitoring and discover functions past espresso, similar to detecting defects in different agricultural merchandise.”
The total examine, referred to as “Comparative evaluation of YOLO fashions for inexperienced espresso bean detection and defect classification,” was revealed within the Nature journal Scientific Studies.
Feedback? Questions? Information to share? Contact DCN’s editors right here.